2024
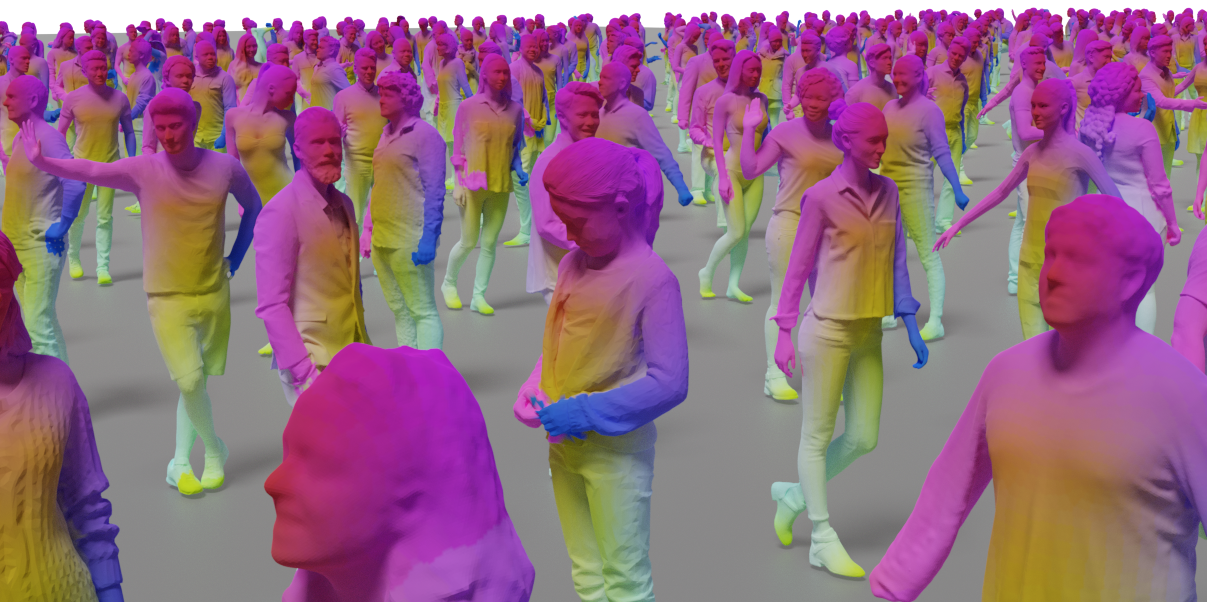
NICP: Neural ICP for 3D Human Registration at Scale
We propose a novel localized Neural Field (LoVD), the first self-supervised task for tuning neural fields (INT), and an efficient (takes less than a minute) scalable registration pipeline (NSR), that works with out-of-distribution data (partial point clouds, clutter, different poses, …).
2023
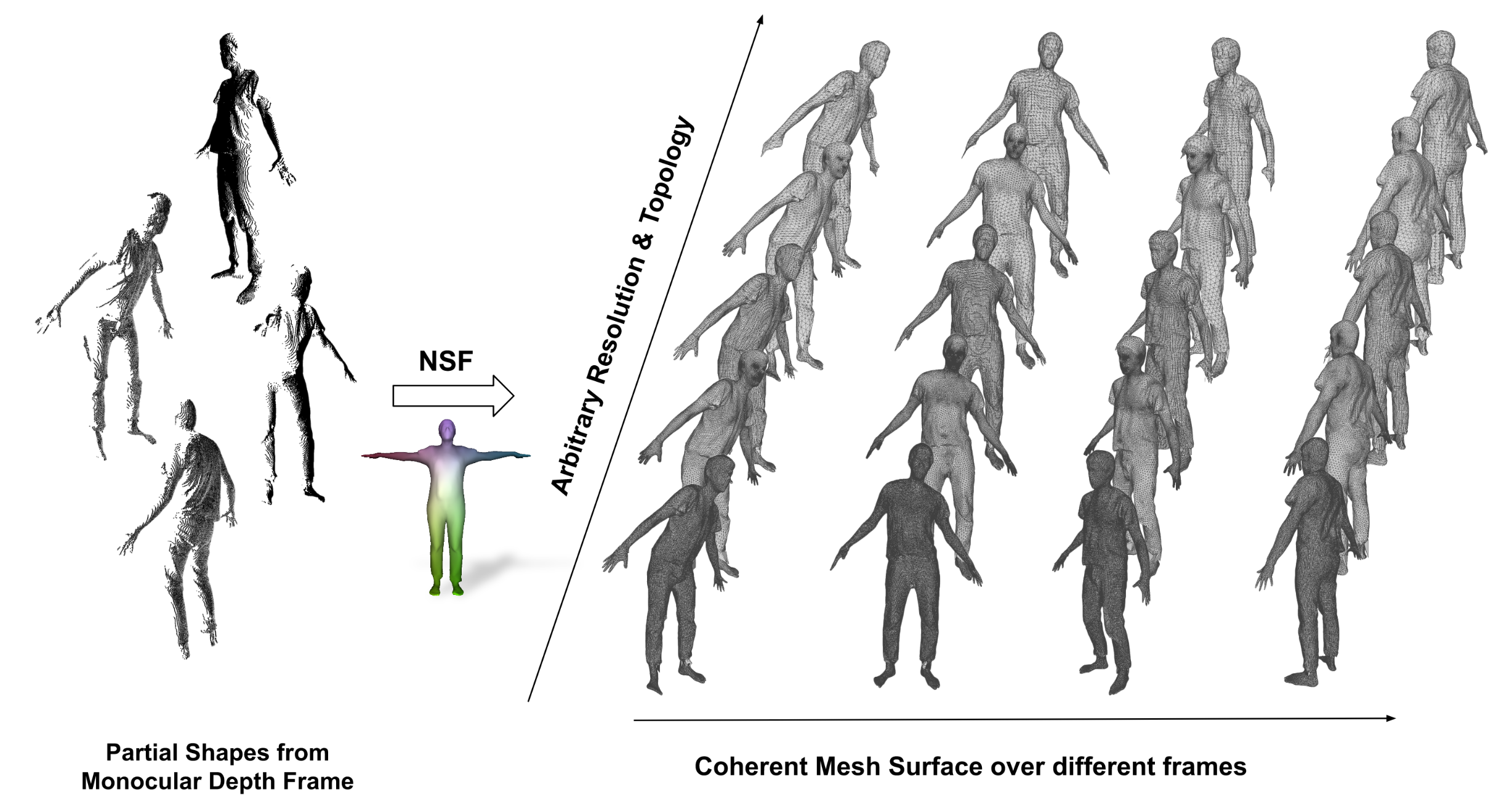
NSF: Neural Surface Fields for Human Modeling from Monocular Depth
The Neural Surface Fields (NSF) defines a Neural Field on the level set of an implicit representation, providing a continuous and flexible function representation on 3D geometries. We apply it in an avatarization pipeline, learning animatable avatars with pose-dependent deformations starting from a sparse set of partial depth views.
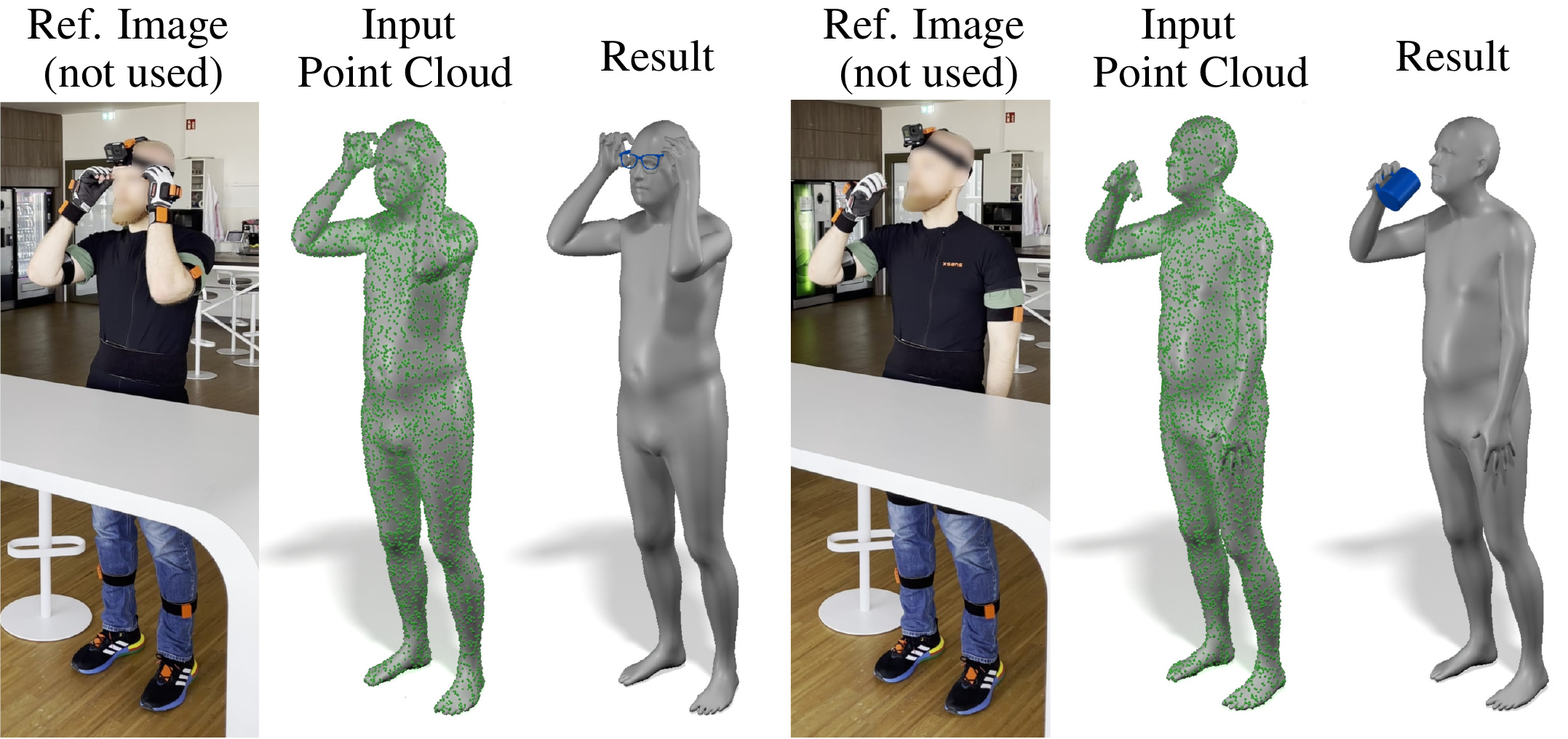
Object pop-up: Can we infer 3D objects and their poses from human interactions alone?
We show that an unorganized 3D human point cloud provides enough information to infer a 3D interacted object, opening new directions in the human-object interaction research. We also analyze the impact of different levels of information and a saliency study about the geometrical features of the input human point cloud.